This article explores some new and emerging applications of text analytics and natural language processing (NLP) in healthcare. Each application demonstrates how HCPs and others use natural language processing to mine unstructured text-based healthcare data and then do something with the results.
Healthcare databases are growing exponentially, and text analytics and natural language processing (NLP) systems turn this data into value. Healthcare providers, pharmaceutical companies and biotechnology firms all use text analytics and NLP to improve patient outcomes, streamline operations and manage regulatory compliance.
In order, we’ll talk about:
- Sources of healthcare data and how much is out there
- Improving customer care while reducing Medical Information Department costs
- Hearing how people really talk about and experience ADHD
- Facilitating value-based care models by demonstrating real-world outcomes
- Guiding communications between pharmaceutical companies and patients
- Even more applications of text analytics and natural language processing in healthcare
- Some more things to think about, including major ethical concerns
NLP in the Healthcare Industry: Sources of Data for Text Mining
Patient health records, order entries, and physician notes aren’t the only sources of data in healthcare. In fact, 26 million people have already added their genetic information to commercial databases through take-home kits. And wearable devices have opened new floodgates of consumer health data. All told, Emerj lists 7 healthcare data sources that, especially when taken together, form a veritable goldmine of healthcare data:
1. The Internet of Things (IoT) think FitBit data)
2. Electronic Medical Records (EMR)/Electronic Health Records (EHR) (classic)
3. Insurance Providers (claims from private and government payers)
4. Other Clinical Data (including computerized physician order entries, physician notes, medical imaging records, and more)
5. Opt-In Genome and Research Registries
6. Social Media (tweets, Facebook comments, message boards, etc.)
7. Web Knowledge (emergency care data, news feeds, and medical journals)
Just how much health data is there from these sources? More than 2,314 exabytes by 2020, says BIS Research. For reference, just 1 exabyte is 10^9 gigabytes. Or, written out, 1EB=1,000,000,000GB. That’s a lot of GB.
But adding to the ocean of healthcare data doesn’t do much if you’re not actually using it. And many experts agree that utilization of this data is… underwhelming. So let’s talk about text analytics and NLP in the health industry, particularly focusing on new and emerging applications of the technology.
Improving Customer Care While Reducing Medical Information Department Costs
Every physician knows how annoying it can be to get a drug-maker to give them a straight, clear answer. Many patients know it, too. For the rest of us, here’s how it works:
- You (a physician, patient or media person) call into a biotechnology or pharmaceutical company’s Medical Information Department (MID)
- Your call is routed to the MID contact center
- MID operators reference all available documentation to provide an answer, or punt your question to a full clinician
Simple in theory, sure. Unfortunately, the pharma/biotech business is complicated. Biogen, for example, develops therapies for people living with serious neurological and neurodegenerative diseases. When you call into their MID to ask a question, Biogen’s operators are there to answer your inquiry. Naturally, you expect a quick, clear answer. At Biogen Japan, any call that lasts more than 1 minute is automatically escalated to an expensive second-line medical directors. Before, Biogen struggled with a high number of calls being escalated because their MID agents spent too long parsing through FAQs, product information brochures, and other resources.
Today, Biogen uses text analytics (and some other technologies) to answer these questions more quickly, thereby improving customer care while reducing their MID operating costs. When you call into their MID, operators use a Lexalytics-built search application that combines natural language processing and machine learning to immediately suggest best-fit answers and related resources to people’s inquiries. MID operators can type in keywords or exact questions and get what they need in seconds. (The system looks like this illustration.) Early testing already shows faster answers and fewer calls sent to medical directors, and the application also helps new hires work at the level of experienced operators, further reducing costs.
Hearing How People Really Talk About and Experience ADHD
The human brain is terribly complicated, and two people may experience the same condition in vastly different ways. This is especially true of conditions like Attention Deficit Hyperactivity Disorder (ADHD). In order to optimize treatment, physicians need to understand exactly how their individual patients experience it. But people often tell their doctor one thing, and then turn around and tell their friends and family something else entirely.
A Lexalytics data scientist used our text analytics and natural language processing to analyze data from Reddit, multiple ADHD blogs, news websites, and scientific papers sourced from the PubMed and HubMed databases. Based on the output, they modeled the conversations to show how people talk about ADHD in their own words.
The results showed stark differences in how people talk about ADHD in research papers, on the news, in Reddit comments and on ADHD blogs. Although our analysis was fairly basic, our methods show how using text analytics in this way can help healthcare organizations connect with their patients and develop personalized treatment plans.
Facilitating Value-Based Care Models by Demonstrating Real-World Outcomes
Our analysis of conversations surrounding ADHD is just one example in the large field of text analytics in healthcare. Everyone involved in the healthcare value chain, including HCPs, drug manufacturers, and insurance companies are using text analytics as part of the drive towards value-based care models.
Within the value-based care model, and outcome-based care in general, providers and payers all want to demonstrate that their patients are experiencing positive outcomes after they leave the clinical setting. To do this, more and more stakeholders are using text analytics systems to analyze social media posts, patient comments, and other sources of unstructured patient feedback. These insights help HCPs and others identify positive outcomes to highlight and negative outcomes to follow-up with.
Some HCPs even use text analytics to compare what patients say to their doctors, versus what they say to their friends, to identify how they can improve patient-clinician communication. In fact, the larger trend here almost exactly follows the push in more retail-focused industries towards data-driven Voice of Customer: using technology to understand how people talk about and experience products and services, in their own words.
Guiding Communications Between Pharmaceutical Companies and Patients
Pharmaceutical marketing teams face countless challenges. These include growing market share, demonstrating product value, increasing patient adherence and improving buy-in from healthcare professionals. Lexalytics customer AlternativesPharma helped those professionals by providing useful market insights and effective recommendations.
Before, companies like AlternativesPharma relied on basic customer surveys and some other quantitative data sources to create their recommendations. Using our text analytics and natural language processing, however, AlternativesPharma was able to categorize large quantities of qualitative, unstructured patient comments into “thematic maps.” The output of their analyses led to research publications at the 2015 Nephrology Professional Congress and in the Journal Néphrologie et Thérapeutiques.
Further, AlternativesPharma helped customers verify assumptions made by Key Opinion Leaders (KOLs) regarding the psychology of patients with schizophrenia. This theory was then documented in collateral and widely communicated to physicians. (Full case study)
More Applications of Text Analytics and Natural Language Processing in Healthcare
The above applications of text analytics in healthcare are just the tip of the iceberg. McKinsey has identified several more applications of NLP in healthcare, under the umbrellas of “Administrative cost reduction” and “Medical value creation”. Their detailed infographic is a good explainer. Click the image (or this link) to read the full infographic on McKinsey’s website.
Meanwhile, this 2018 paper in The University of Western Ontario Medical Journal titled “The promise of natural language processing in healthcare” dives into how and where NLP is improving healthcare. The authors, Rohin Attrey and Alexander Levitt, divide healthcare NLP applications into four categories. These cover NLP for:
- Patients – including teletriage services, where NLP-powered chatbots could free up nurses and physicians
- Physicians – where a computerized clinical decision support system using NLP has already demonstrated value in alerting clinicians to consider Kawasaki disease in emergency presentations
- Researchers – where NLP helps enable, empower and accelerate qualitative studies across a number of vectors
- Healthcare Management – where patient experience management is brought into the 21st-century by NLP used on qualitative data sources
Next, researchers from Sant Baba Bhag Singh University explored how healthcare groups can use sentiment analysis. The authors concluded that using sentiment analysis to examine social media data is an effective way for HCPs to improve treatments and patient services by understanding how patients talk about their Type-1 and Type-2 Diabetes treatments, drugs, and diet practices.
Finally, market research firm Emerj has written up a number of NLP applications for hospitals and other HCPs, including systems from IQVIA, 3M, Amazon and Nuance Communications. These applications include improving compliance with industry standards and regulations; accelerating and improving medical coding processes; building clinical study cohorts; and speech recognition and speech-to-text for doctors and healthcare providers.
Some More Things to Consider: Data Ethics, AI Fails, and Algorithmic Bias
If you’re thinking about building or buying any data analytics system for use in a healthcare or biopharma environment, here are some more things you should be aware of and take into account. All of these are especially relevant for text analytics in healthcare.
First: According to a study from the University of California Berkeley, advances in artificial intelligence (AI) have rendered the privacy standards set by the Health Insurance Portability and Accountability Act of 1996 (HIPAA) obsolete. We investigated and found some alarming data privacy and ethics concerns surrounding AI in healthcare.
Read – AI in Healthcare: Data Privacy and Ethics Concerns
Second: Companies with regulatory compliance burdens are flocking to AI for time savings and cost reductions. But costly failures of large-scale AI systems are also making companies more wary of investing millions into big projects with vague promises of future returns. How can AI deliver real value in the regulatory compliance space? We wrote a white paper on this very subject.
Read – A Better Approach to AI for Regulatory Compliance
Third: The “moonshot” attitude of big tech companies comes with huge risk for the customer. And no AI project tells the story of large-scale AI failure quite like Watson for Oncology. In 2013, IBM partnered with The University of Texas MD Anderson Cancer Center to develop a new “Oncology Expert Advisor” system. The goal? Nothing less than to cure cancer. The result? “This product is a piece of sh–.”
Read – Stories of AI Failure and How to Avoid Similar AI Fails
Fourth: “Bias in AI” refers to situations where machine learning-based data analytics systems discriminate against particular groups of people. Algorithmic bias in healthcare AI systems manifests when data scientists building machine learning models for healthcare-related use cases train their algorithms on biased data from the start. Societal biases manifest when the output or usage of an AI-based healthcare system reinforces societal biases and discriminatory practices.
Read – Bias in AI and Machine Learning: Sources and Solutions
Improve Your Understanding: What Are Text Analytics and Natural Language Processing?
In order to put any tool to good use, you need to have some basic understanding of what it is and how it works. This is equally true of text analytics and natural language processing. So, what are they?
Text analytics and natural language processing are technologies for transforming unstructured data (i.e. free text) into structured data and insights (i.e. dashboards, spreadsheets and databases). Text analytics refers to breaking apart text documents into their component parts. Natural language processing then analyzes those parts to understand the entities, topics, opinions, and intentions within.
The 7 basic functions of text analytics are:
- Language Identification
- Tokenization
- Sentence Breaking
- Part of Speech Tagging
- Chunking
- Syntax Parsing
- Sentence Chaining
Natural language processing features include:
Categorization (topics and themes)
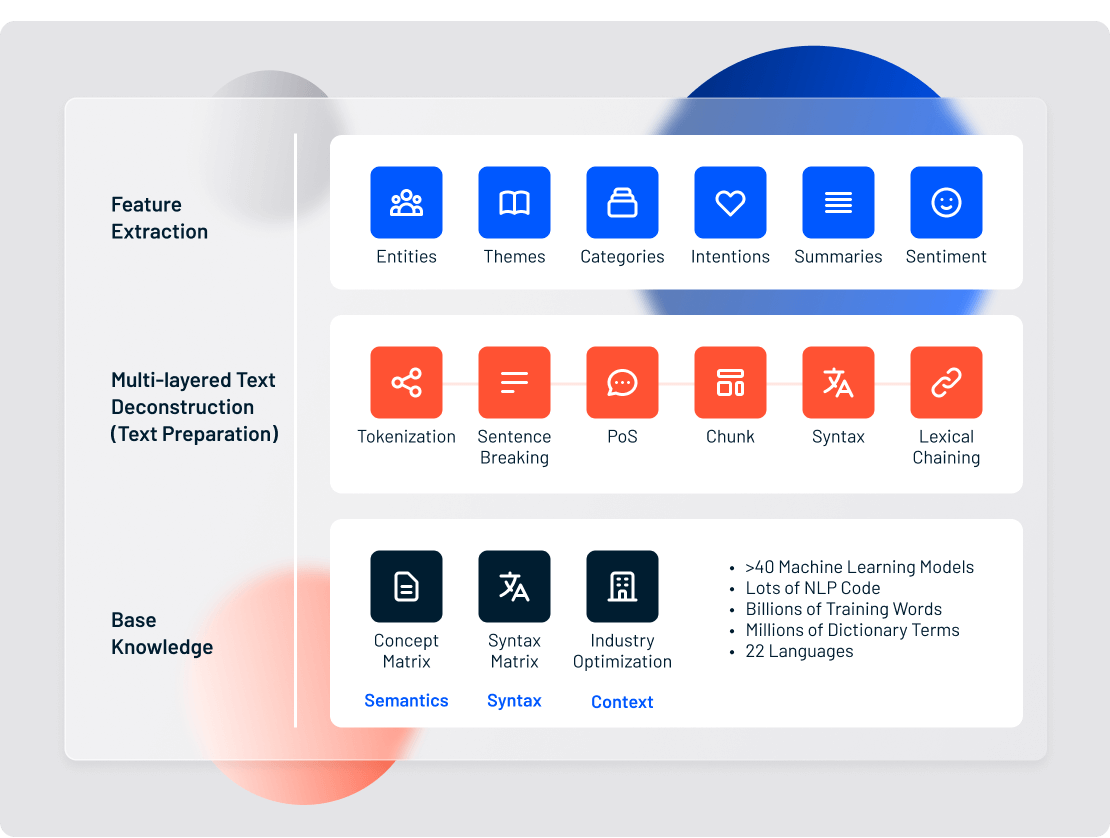
Beyond the basics, semi-structured data parsing is used to identify and extract data from medical, legal and financial documents, such as patient records and Medicaid code updates. Machine learning improves core text analytics and natural language processing functions and features. And machine learning micromodels can solve unique challenges in individual datasets while reducing the costs of sourcing and annotating training data.